Chat GPT and Bus Scheduling
This week, I aim to provide an introductory overview of how ChatGPT can assist with bus scheduling.
During a recent demonstration at the American Bus Association Marketplace, attended by a group specializing in intercity line-runs, I suggested exploring the optimal departure time for a bus to maximize its passenger carrying potential. This article summarizes that presentation.
Background
Many of the companies run scheduled services that operate just one trip in each direction per day, covering very large distances, often over 400 miles. Some stops are large cities, while others are much smaller urban areas.
The start location of a trip is typically determined by where the operator had a physical base, and not necessarily by the size of the city.
The question posed was "what's the best time for the bus to start this trip?"
To start, we know (or at least assume) that ridership is a function of:
Population (bigger cities = bigger potential market).
Departure time (daytime departures will generally pick up more customers than departures between 1am and 4am).
There are other inputs of course, but I'll stick to these to get going and we'll look at adding the other inputs later.
Our Route - Harborview to Starfield
Using a bunch of random locations, populations and journey times, we can begin to formulate a route. I kept the total run time compliant for one US based driver to do the shift, assuming minimal deadhead time.
Population and Travel Time
The details of population and run times for our fictitious route are presented in the table below. The start city population, Harborview, isn't the biggest city, but it is where our bus company is located.
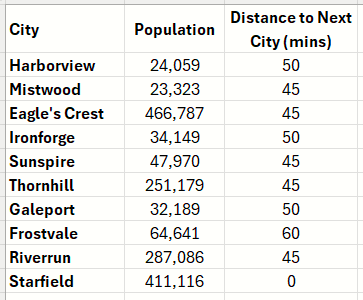
On this route, the bus will end at Starfield where it will have access to cleaning and refuel only. The biggest population center is almost 2 hours into the route from Harborview, with Riverrun and Starfield being the the next biggest as the last two places served.
Departure Time Weighting
Next, we know that certain times of day stand a better chance of picking up customers than others. Taking a real-world example, a 2pm Indianapolis to Chicago run is more likely to pick up customers than a 2am run.
Let's make a table to reflect these differences. A value of 100 means max customer potential at that time. A value near 0 means no potential. These values are anecdotal and will vary depending on market and distance from origin and destination:
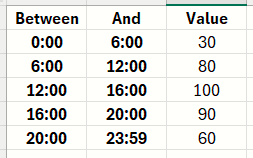
With these 3 pieces of information (population, run time and departure time weighting), we can attempt our first query with ChatGPT.
The Query (or Prompt):
The query quality is important to direct ChatGPT in the area you need. Here's what I used:
I'm an intercity bus operator who operates one trip per day in each direction of the list of cities I provide in the following tables. [Copy/paste the tables]
The first table has the sequential list of cities, their populations and journey times to the next city.
The second table has weightings that need to be considered for the fact that not all departure times are equal.
Using this information and assume I want to optimize for maximum passenger carrying potential, provide a schedule in both directions.
The more constraints or parameters that are listed, the less likely GPT is going to be successful at providing an output at least in the first couple of rounds.
Breaking it down into smaller incremental steps may increase the chance of success.
The Analysis:
Assuming we get a good hit, we see ChatGPT generate the thought process - and it makes reasonable sense. Here is the initial commentary I got with the above prompts:
For the outbound trip, we would aim to pass through Eagle's Crest, Thornhill, Riverrun, and Starfield during the peak hours, as they are the most populous. To do this, we'll work backward from the peak time to determine the latest possible departure time from Harborview.
For the inbound trip, we'll do the reverse, starting from Starfield and aiming to pass through the populous cities during the peak time frame.
(Note the GPT output above may not be visible on the emailed version of the LinkedIn newsletter (I'm not quite sure why). Consider clicking through the newsletter to view on your web browser.)
GPT continues to come up its optimized solution as follows:
Given that the peak window is 4 hours long, we should aim to start the outbound trip around 6:20 AM to ensure we're traveling through the most populous cities during the peak times. This allows for travel through most cities during the 12:00 - 16:00 window, which has the highest value of 100.
For the inbound trip, if we want to pass through the most populous cities during the 16:00 - 20:00 window (which has a value of 90), we need to consider the 6 hours and 30 minutes of travel time. To optimize for passenger potential, we would want to start the inbound trip at Starfield around 9:30 AM.
Let's draft the schedules based on these starting times and the travel times between cities.
Here are the optimized schedules:
City Departure Time
Starfield 9:30
Riverrun 10:15
Frostvale 11:15
Galeport 12:05
Thornhill 12:50
Sunspire 13:35
Ironforge 14:25
Eagle's Crest 15:10
Mistwood 15:55
Harborview 16:45
City Departure Time
Harborview 6:20
Mistwood 7:10
Eagle's Crest 7:55
Ironforge 8:40
Sunspire 9:30
Thornhill 10:15
Galeport 11:00
Frostvale 11:50
Riverrun 12:50
Starfield 13:35
It's a decent starting point. There were some generalizations made (picking the biggest cities to fall within the best time bands, and assuming the rest would fall into place). But this is still a far better educated guess than "I'll start the trip at 6am".
Refinements:
You might want to ask ChatGPT for more information, like:
"Provide a visual representation of how you get to this schedule". GPT will often generate basic graphs or diagrams illustrating the computation.
Re-optimize instead of using generalizations for big populations and best departure times. "Please optimize for all locations across all times and provide the optimal journey start time." This would be useful if in doubt that method taken is not sufficient.
"What are the limitations of the method used to come up with this solution?" We don't know what we don't know. Ask ChatGPT to warn you of the limitations of this particular optimization. Ask it to regenerate in a way that reduces those limitations.
"What instructions could improve the optimization of the schedule?" Particularly if you're not data savvy, this may help you to request a more stronger constructed schedule.
Taking it further:
While I used population, duration and departure time as factors to consider, there are others. You may want a schedule that maximizes revenue versus maximizes passenger counts. Issues such as arrival time at destination and differences in cities where people are more likely to take a bus are also important factors to consider.
You might create additional tables and ask GPT to consider these. For example:
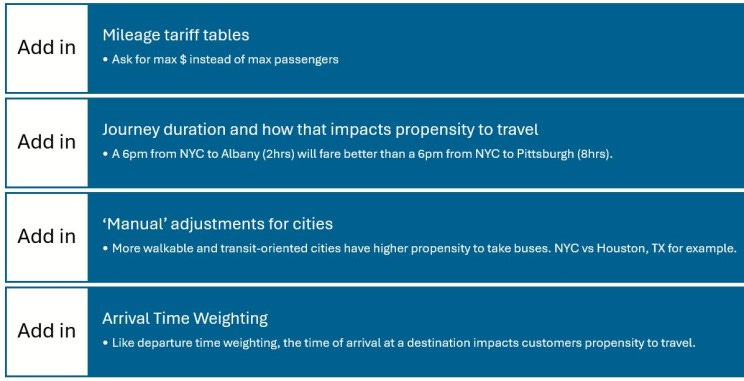
While I could demonstrate almost no limit of possibilities and tweaks, each company will have different priorities. I would encourage you to play around. You don't need to be technical. You don't need to be apologetic for putting in a bad prompt. You can tell it that it didn't give you what you were expecting and it might ask questions to help refine your query.
Beyond the intercity schedule
While I've had some success in generating 'optimal' schedules using ChatGPT, the more traditional scheduling tasks of bus blocking, run-cutting and roster generation tend to push it over the edge, particularly when you go beyond a couple of buses in peak vehicle requirements (PVR).
For transit scheduling (higher frequency routes, PVR's etc.) I've not been able to get far with ChatGPT. The traditional electronic scheduling tools provided by the like of Optibus , Trapeze Group or GIRO have at their core the algorithms and power to run thousands of iterations in a short time with dozens of parameters, rules and limitations applied. ChatGPT doesn't come close to being able to consider all these at once. You'll see mostly due to time-outs, which I presume, is for the processing needed to generate the schedulers products.
However ChatGPT can still help with solution development that use the outputs of scheduling software. A few examples include:
Generating a schedule of cleaners, fuellers based on bus run-in and run-out times.
Help with staff planning to get to the peak driver requirement for an upcoming roster / service increase.
Help with generating a vacation roster for the year.
Help with deciding when to put ground support (what's the best 8 hours to have ground staff active, given buses per hour, passengers per hour etc) on the ground.
Overall, ChatGPT can help you get started with the crossroads of data and scheduling - particularly for non-data oriented folk. It can help present outcomes, constraints and challenges in plain language. It's a low cost way of getting acquainted with scheduling challenges which may give you a better steer on what exactly you may need for your business beyond ChatGPT in terms of scheduling capabilities.
Nevertheless, I hope this has given you some food for thought in how, beyond language generation, ChatGPT can take numbers and data and help unlock the potential of these in your transit and intercity business.